Deep learning for 3D particle field imaging
Spring 2021
Master Semester Project
Project: 00409
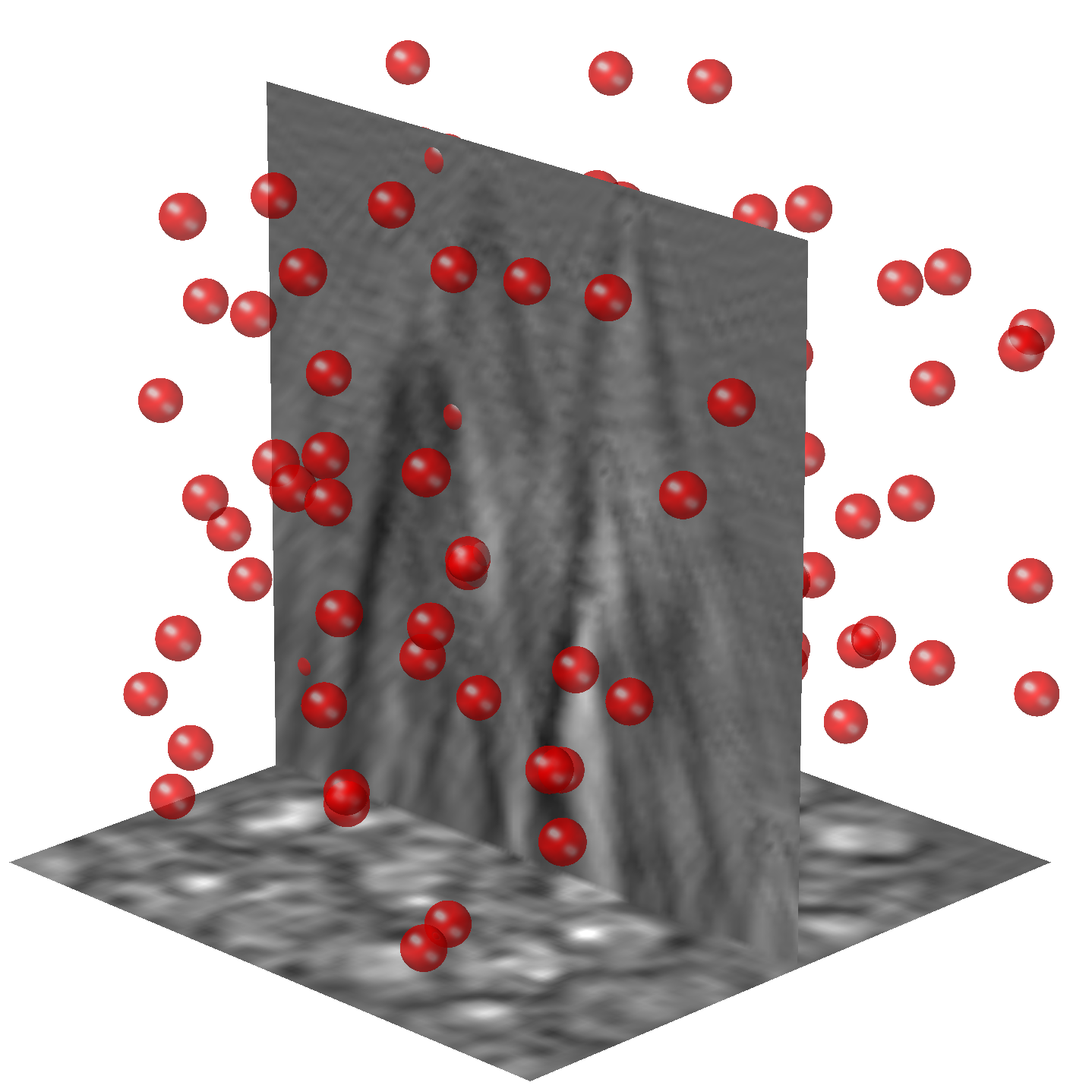
Particle fields include a large range of samples of interest, such as bubbles, droplets, or biological cells.
To obtain a three-dimensional (3D) volume of such fields, one popular method involves in-line digital holography (DH).
In this imaging modality, the particle field is illuminated with an incident field (light) so that multiple scattering and diffraction occur. The resulting field is then holographically recorded.
From a single two-dimensional (2D) DH image, computational methods are able to recover the particles within a 3D volume. When the density of particles and/or the depth of field are large, the reconstruction task becomes too difficult for conventional methods.
During this project, the student will implement and train a neural network to recover particles within a 3D volume from a 2D image. The programming language is Python (Pytorch). Based on an existing code in Matlab, the student will also implement the physical model which describes the wave propagation in Pytorch. The required skills are prior knowledge of deep learning, proficiency in coding in Pytorch. The student should be able to learn the basics of wave propagation and optics during the project.
During this project, the student will understand the physical model of an imaging modality, learn how to conduct a complete project with deep learning, and learn how to use a physical model combined with deep learning.
References Tahir, W., Kamilov, U. S., & Tian, L. (2019). Holographic particle localization under multiple scattering. Advanced Photonics, 1(3), 036003.
During this project, the student will implement and train a neural network to recover particles within a 3D volume from a 2D image. The programming language is Python (Pytorch). Based on an existing code in Matlab, the student will also implement the physical model which describes the wave propagation in Pytorch. The required skills are prior knowledge of deep learning, proficiency in coding in Pytorch. The student should be able to learn the basics of wave propagation and optics during the project.
During this project, the student will understand the physical model of an imaging modality, learn how to conduct a complete project with deep learning, and learn how to use a physical model combined with deep learning.
References Tahir, W., Kamilov, U. S., & Tian, L. (2019). Holographic particle localization under multiple scattering. Advanced Photonics, 1(3), 036003.
- Supervisors
- Thanh-An Pham, thanh-an.pham@epfl.ch, BM 4.140
- Michael Unser, michael.unser@epfl.ch, 021 693 51 75, BM 4.136