1-Bit Compressed Imaging
Principal Investigator: Aurélien Bourquard
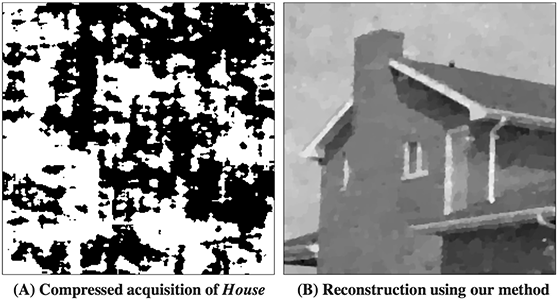
Summary
Based on the compressed-sensing (CS) theory, we propose an efficient approach to acquire images in a compressed binary form, and propose the reconstruction algorithm that is optimized for this type of data. Our global strategy relies on
Introduction
Compressed sensing is a recent paradigm that allows one to substantially reduce the amount of data to be acquired as compared to conventional sampling strategies. The key principle is to compress the information before it is captured, which is especially beneficial when the acquisition process is expensive in terms of time or hardware. In this project, we reduce the amount of image data by quantizing measurements to one single bit per pixel. The original image is then recovered through numerical reconstruction using an appropriate algorithm.
Main Contribution
We propose a new technique to acquire images in compressed form. Our forward model is physically realistic, and produces binary measurements through random convolution (as introduced by J. Romberg) followed by 1-bit quantization. Adapting the 1-bit CS paradigm introduced by P. Boufounos to our model, and making it suitable for convex optimization, we have developed an efficient reconstruction algorithm that only involves fast Fourier transforms. Our current research deals with an improved setting where the compressed data consists in a set of several such acquisitions.
Collaboration: Michael Unser
Period: 2008-ongoing
Funding: Grant application pending
Major Publications
- , , , Optical Imaging Using Binary Sensors, Optics Express, vol. 18, no. 5, pp. 4876–4888, March 1, 2010.