Steerable Wavelets |
Investigators: Nicolas Chenouard |
|
Summary: We investigate a functional framework for the design of steerable wavelet frames that are both signal and application adaptive. The cornerstone of our approach is the generalized Riesz transform which brings steerability to a primary isotropic wavelet transform and can be shaped according to the application, in particular, by using optimization and learning techniques. We investigate both 2D and 3D steerable wavelets frames, which opens the way to new image-processing techniques for biomedical data. |
|
The main goal of this work is to set the foundations of a general continuous-domain framework for designing steerable, multiscale, reversible signal transformations (a.k.a. wavelet frames) in multiple dimensions, and to design new 2D and 3D image-processing algorithms taking advantage of it. We have given a complete functional description of these generalized Riesz wavelet transforms and we have derived their steering equations. Moreover, we have established a link between the proposed framework and the popular steerable wavelet pyramid in 2D. In a number of applications (e.g., image denoising, image reconstruction from edges) we have illustrated the potential of the new transforms, in particular when using learning techniques to adapt the frame to the signal. A prominent application of these transforms is the extension of the analytic signal to 2D and 3D through the use of the Riesz wavelet transform. |
|
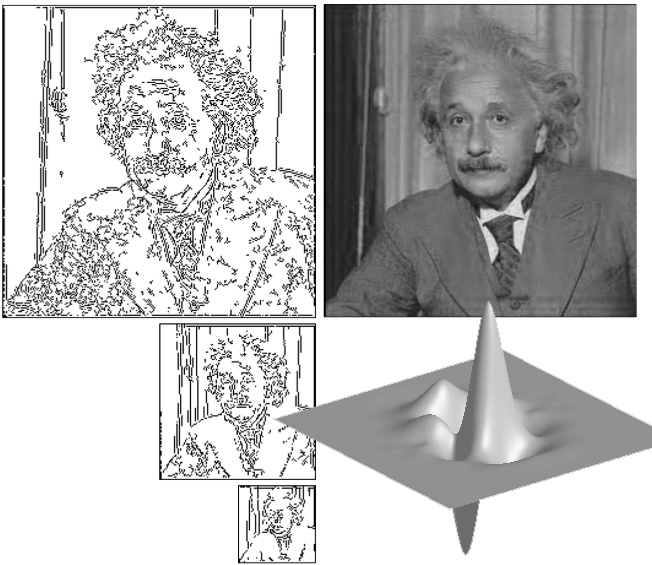 |
Left: Wavelet primal sketch of the "Einstein" image; Right: Reconstruction from the primal sketch; Bottom right: Marr wavelet (real part). |
We have built and characterized a functional framework for the design of steerable wavelet frames in multiple dimensions. This novel framework relies on the proposed generalized Riesz wavelet transform of high order and is parameterized by a shaping matrix that offers a great flexibility for the design of custom steerable frames.
A fast filterbank implementation has been described for the proposed Riesz wavelet transforms, as well as algorithms for efficiently steering basis functions. In particular, the proposed framework has allowed us to design the first 3D steerable wavelet transform. We are investigating further multiple aspects of the steerable wavelet design such as best-basis learning for a given application.
We have proposed a multiscale monogenic transform that relies on a particular instance of the proposed steerable wavelet family. This allows us to analyze the local orientation of the signal and to derive an extension of the analytical signal in 2D and in 3D. |
|
Collaborations: Michael Unser, Brigitte Forster (TUM) |
|
Past Investigators: Dimitri Van De Ville |
|
|
Funding: SNSF project granted |
|
|
|
|