Thresholding strategies for image denoising with an application to fMRI statistical analysis
2005
Master Diploma
Project: 00118
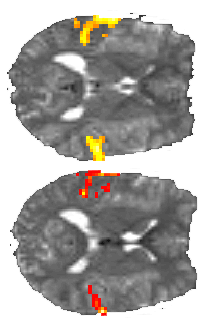
Wavelet image denoising has gained a lot of attention in the past fifteen years, in particular thanks to the strong influence of statisticians like D. Donoho. We propose to apply the Stein unbiased risk estimate (SURE) approach to wavelet thresholding and extend it to spatially correlated models, redundant wavelet transforms, and biorthonormal wavelet transforms.
In a second part of the project, we propose to analyze how it is possible to apply these approaches to the statistical detection of activations in Functional magnetic resonance imaging (fMRI), which is a fast-developing technique for studying physiological processes in the brains of conscious human subjects.
The project will thus be roughly be decomposed into three uneven parts:
1) a theoretical analysis of Stein's risk theory to vector data and potential numerical implementations of its minimization;
2) implementation of several image denoising algorithms and comparison of the results with the up-to-date literature. Several wavelet bases will be tested in the processing;
3) analysis of fMRI detection process, reformulated as a denoising problem following a recently developped approach in our group.
In a second part of the project, we propose to analyze how it is possible to apply these approaches to the statistical detection of activations in Functional magnetic resonance imaging (fMRI), which is a fast-developing technique for studying physiological processes in the brains of conscious human subjects.
The project will thus be roughly be decomposed into three uneven parts:
1) a theoretical analysis of Stein's risk theory to vector data and potential numerical implementations of its minimization;
2) implementation of several image denoising algorithms and comparison of the results with the up-to-date literature. Several wavelet bases will be tested in the processing;
3) analysis of fMRI detection process, reformulated as a denoising problem following a recently developped approach in our group.
- Supervisors
- Thierry Blu, thierry.blu@epfl.ch, 021 693 51 72, BM 4.134
- Michael Unser, michael.unser@epfl.ch, 021 693 51 75, BM 4.136