Restoring axial resolution using a 2D/3D deep convolution neuronal network
Spring 2017
Master Semester Project
Project: 00327
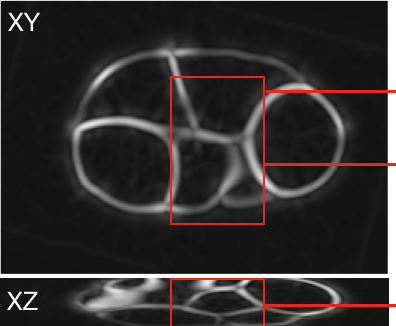
In 3D fluorescence microscopy, the axial resolution (axis Z) is often lower than the lateral resolution (optical plane XY). This non-isotropic resolution penalizes the global resolution of the biological structures. The conventional way to expand the size is to interpolate in the Z axis which create blurry 3D images without recovering any structure.
In this project, we propose to recover the axial resolution based on the deep learning method. We propose a 2D strategy by patches to build a reasonable system. The learning will be performed in the 2D XY plane (higher resolution) than the reconstruction in performed in the 2D XZ or/and in the YZ planes. The learning with be done using a deep convolution neuronal network (CNN) in particular using a multiresolution approach with the U-net architecture. This recent architecture has demonstrated very good results in many fields.
- Supervisors
- Daniel Sage, daniel.sage@epfl.ch, 021 693 51 89, BM 4.135
- Michael Unser, michael.unser@epfl.ch, 021 693 51 75, BM 4.136
- Kyong Jin, kyong.jin@epfl.ch