Fast Structured Illumination Microscopy by Reducing the Number of Images Required
2022
Master Diploma
Project: 00426
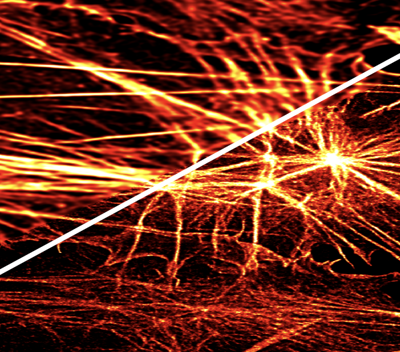
Structured Illumination Microscopy (SIM) is a method that was developed to overpass the optical
diffraction limit by a factor of 2, i.e., up to ~120 nm. It relies on interference patterns
that are generated by projecting a periodic grating onto the image plane of the microscope.
Due to the modulation property of the Fourier Transform, this grating shifts the high frequency
components of the sample to the bandwith of the optical system. Then, dedicated algorithms
are deployed to unmix these high frequencies and reconstruct a super-resolved image.
At BIG, there are ongoing projects to design algorithms and regularizers for SIM. Fasten
the acquisition by reducing the number of raw images is a priority in recent years.
The goal of the project is to implement a solver for SIM reconstruction using only 4 images (rather than the standard 9). There is no direct formula for the reconstruction from 4 images, and different methods like inverse problems and deep learning are being investigated. Finally, the calibration of the system is another challenging issue to address to obtain competitive results in real applications.
The student will take part in the reconstruction of the super resolution image, parting from datasets provided by BIOP. The tasks include: 1) The study of the mathematical formulation of SIM, 2) Posing and solving the inverse problem with a reduced number of raw data, and 3) Investigating deep learning methods that can further correct reconstruction artifacts. Through this project, the student will gain deep knowledge of SIM, Fourier domain image processing, inverse problems, and deep learning, with an application on super-resolution microscopy. The project will be directed both by the LIB-EPFL (Prof. Unser) and by Emmanuel Soubies (CNRS-IRIT-Toulouse).
The goal of the project is to implement a solver for SIM reconstruction using only 4 images (rather than the standard 9). There is no direct formula for the reconstruction from 4 images, and different methods like inverse problems and deep learning are being investigated. Finally, the calibration of the system is another challenging issue to address to obtain competitive results in real applications.
The student will take part in the reconstruction of the super resolution image, parting from datasets provided by BIOP. The tasks include: 1) The study of the mathematical formulation of SIM, 2) Posing and solving the inverse problem with a reduced number of raw data, and 3) Investigating deep learning methods that can further correct reconstruction artifacts. Through this project, the student will gain deep knowledge of SIM, Fourier domain image processing, inverse problems, and deep learning, with an application on super-resolution microscopy. The project will be directed both by the LIB-EPFL (Prof. Unser) and by Emmanuel Soubies (CNRS-IRIT-Toulouse).
- Supervisors
- Daniel Sage, daniel.sage@epfl.ch, 021 693 51 89, BM 4.135
- Emmanuel Soubies, emmanuel.soubies@irit.fr, IRIT Toulouse
- Michael Unser, michael.unser@epfl.ch, 021 693 51 75, BM 4.136