Diffusion Models for Magnetic Resonance Imaging Reconstruction
On going
Master Diploma
Project: 00462
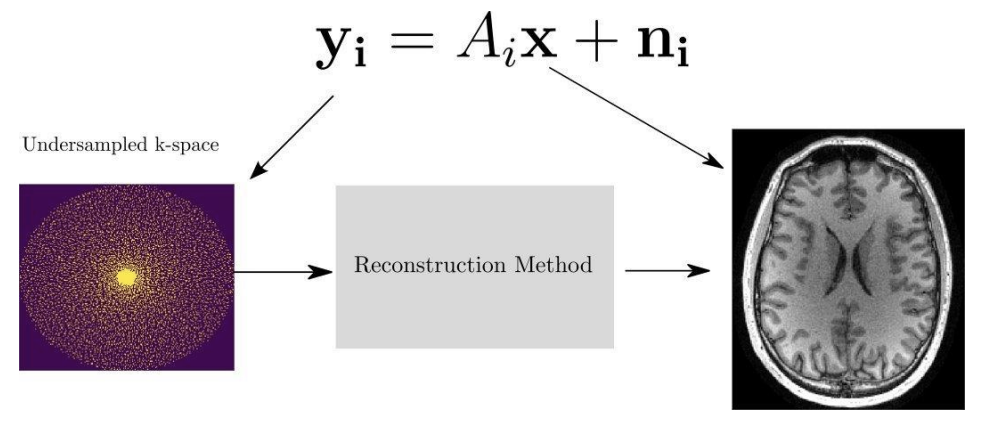
Magnetic Resonance Imaging (MRI) is a well-established medical modality for imaging the human brain and body, capable of producing high resolution images with many different soft tissue contrasts without ionizing radiation. However, as MRI scans are slow due to the physics of acquisition, clinical scans are accelerated by using only incomplete measurements of the Fourier spectrum (or k-space) of the desired image. Consequently, algorithms for reconstructing MRI images with incomplete measurement data have been and are currently of great importance for clinical imaging. As with many computational imaging tasks, machine learning models are now the state of the art for MRI reconstruction, where deep neural networks are trained to act as priors within optimization algorithms using datasets where the acquisitions have been fully sampled (see Figure below). Recently, diffusion models have emerged as an extremely effective way to encode image priors, being used successfully for image generation, image restoration, and image reconstruction.
In this project, we would like to:
• Train and compare several baseline, existing 2D diffusion model reconstructions to our
current in-house DL reconstruction method.
• Investigate new diffusion model architectures and reconstruction frameworks and compare
these to the baselines.
• Assess the feasibility of a 3D diffusion reconstruction for MRI (never assessed so far to our
knowledge).
- Supervisors
- Thomas Yu
- Gian Franco Piredda
- Mehrsa Pourya