Investigation of the multiscale (wavelet-domain) statistics of natural and biomedical images
Spring 2011
Master Diploma
Project: 00228
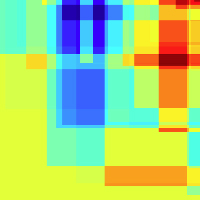
The property that wavelets provide sparse representations of images is exploited in many algorithms for image reconstruction from incomplete / noisy data. We like to view this sparsity as a manifestation of the fact that the statistics of images are non-Gaussian. The goal of this project is to investigate and quantify the non-Gaussian character of images by estimating probability density functions, cumulants and joint dependencies both across wavelets channels (directions) and scale. The ultimate goal is to come up with a parametric statistical model that can be matched to experimental data and used as a practical image prior.
A crucial question is whether or not our recently-constructed classes of sparse stochastic processes is rich enough to explain the type of dependencies and scale evolution observed in real images. This also requires the development of corresponding statistical estimators.
Relevant paper: http://bigwww.epfl.ch/preprints/unser1003p.pdf
A crucial question is whether or not our recently-constructed classes of sparse stochastic processes is rich enough to explain the type of dependencies and scale evolution observed in real images. This also requires the development of corresponding statistical estimators.
Relevant paper: http://bigwww.epfl.ch/preprints/unser1003p.pdf
- Supervisors
- Pouya Dehghani Tafti, 351 35, BM 4.142
- Michael Unser, michael.unser@epfl.ch, 021 693 51 75, BM 4.136